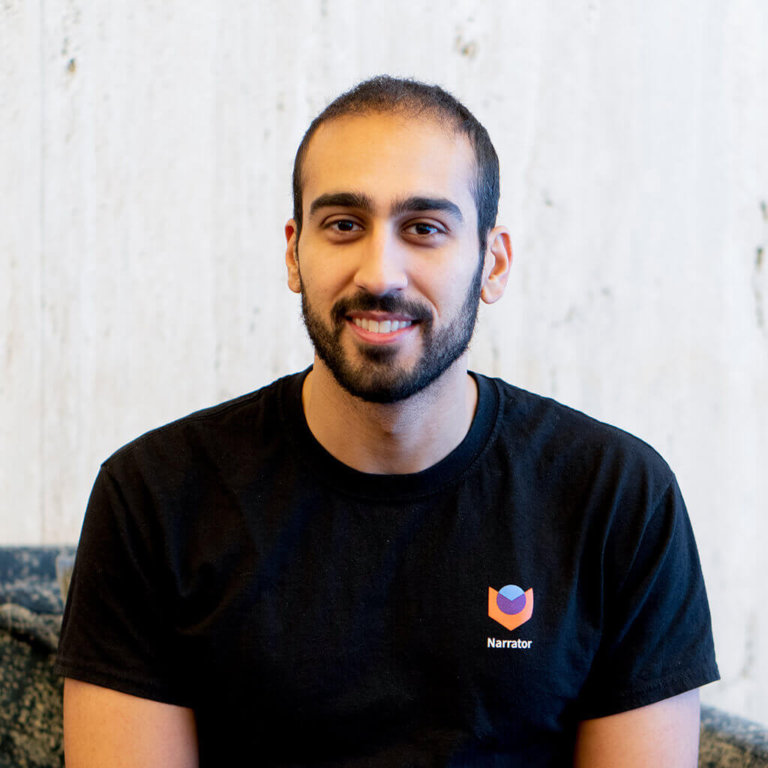
Startups today face tough competition. Around 20% fail within the first year, over 50% fail after five years and over 70% fail after 10. Ahmed Elsamadisi knows these statistics all too well. The robotics graduate from Cornell University was forced to lay off half of the team at his database analysis software company Narrator when the product failed to take off.
After some rethinking and reworking, the company has turned around and found success at Y Combinator, raising US$7.4 million.
Elsamadisi started his career at Cornell’s Autonomous Systems Laboratory focusing on human-robot interaction and building algorithms for autonomous cars. He then moved on to focus on building human exoskeletons — think of Iron Man but made of rubber. He then joined WeWork and grew its data team and built their data infrastructure.
Seeing the success with data analysis at WeWork, he worked to bring this knowledge to the world. From a simple Universal Data Model, he founded his own company to help startups use this approach so they could understand customer behaviour better. Below we speak to Forbes 30 under 30 (Enterprise Technology) icon about his time at Cornell University, Narrator and his future plans:
Walk us through what Narrator does in terms of database analysis. How is this helping companies make decisions based on data?
It enables companies to move much faster, make more decisions and have data be part of their daily lives. The innovation of Narrator is that we have figured out a way to standardise every company’s data, independent of industry or sector into a single table.
That single table can then answer any question. With this superpower, we were able to copy paste analyses from one company to another. From there, we then slowly built a library of analyses that our companies can instantly run.
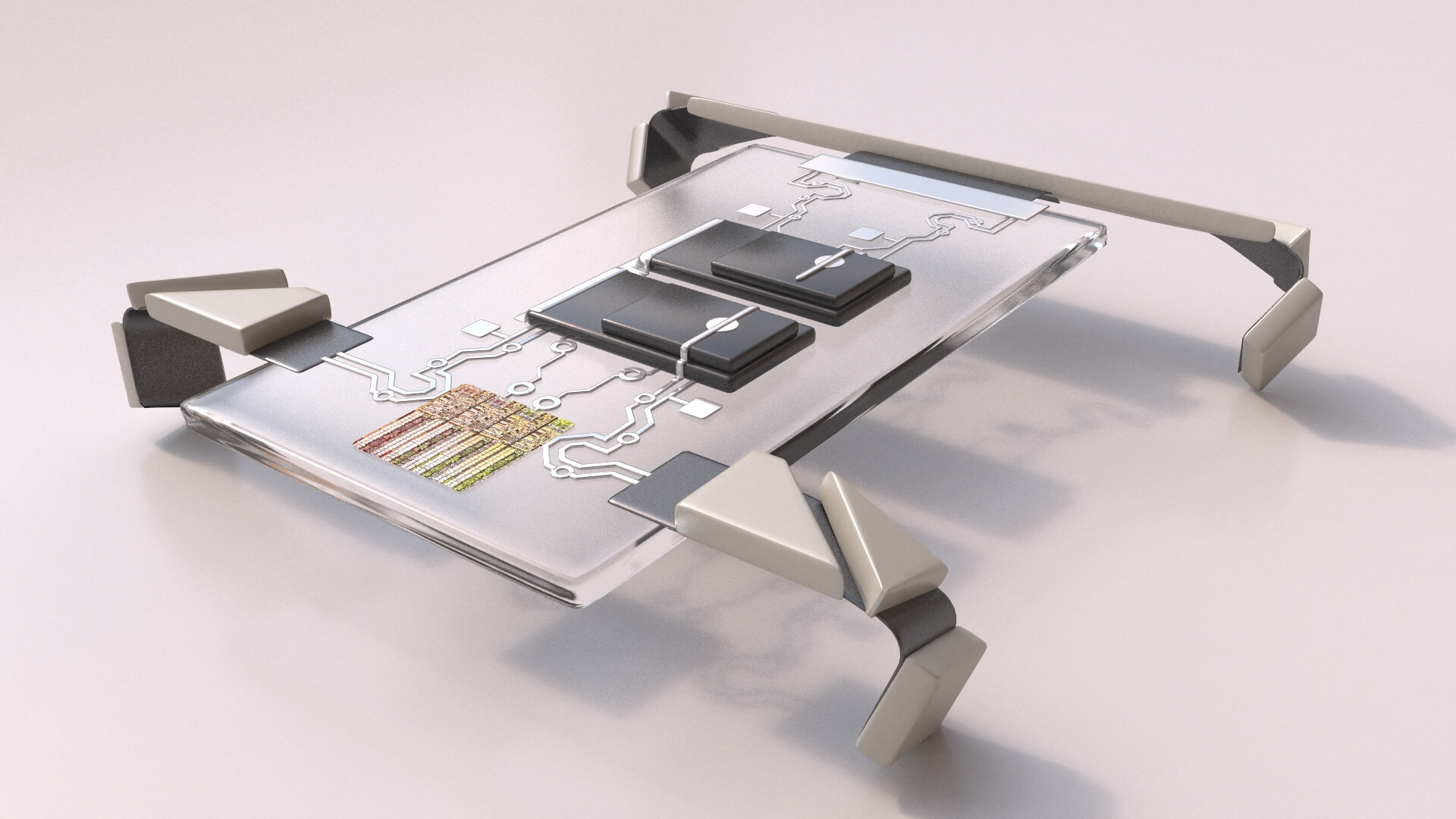
This handout computer generated imaged from a 3D software obtained on August 26, 2020 and released by Cornell University/Christoph Hohmann shows a microscopic four-legged robot. – Scientists have created an army of microscopic four-legged robots too small to see with the naked eye that walk when stimulated by a laser and can be injected into the body through hypodermic needles, a study said on August 26, 2020.
Cornell University is one of the few top tier engineer schools with other top tier schools in it. Source: Christoph Hohmann/AFP
Why did you choose to pursue your Bachelor’s in Robotics at Cornell University?
Cornell University is one of the few top tier engineer schools with other top tier schools in it. This allowed me to focus on engineering but still take classes about business, psychology, communication, leadership and more from incredible professors.
I started out with only knowing three kinds of engineering: mechanical, civil and chemical. As I learned about all the different fields in engineering, I fell in love with how the human minds work. Robotics was a way to replicate human thoughts and decisions with computers.
This excited me beyond anything. I began working in a robotics lab and I found myself more passionate about that research than my classes, so I doubled down on robotics.
AI, cloud and blockchain are all making big waves. How does Narrator use them?
We currently use AI to help write our analysis. Ours can interpret the plots, clusters, detect consistencies, find trends in the data and so much more. I believe if AI is implemented right, users shouldn’t be able to know if the conclusions were made by a computer or by a human. This AI is real, not just marketing.
I find that the industry that talks a lot about using AI/ML are just marketing and chat bots. That is the reason you won’t see AI in any of our branding (outside our domain).
How do you use the knowledge and skills gained at Cornell University in Narrator?
I studied decision-making and used a lot of the algorithms I have learned in my daily work. That being said, I think the more impactful learning was how to approach and solve problems. So much of what we do comes from my ability to take a problem and tie it to a theory with mathematical principles.
I also leverage my mechanical synthesis learning to better approach designing our product. I use my leadership classes to innovate company organisation, and my communication learning to align, motivate and inspire my team. I think I can reference something I learned at Cornell University every day in my job.

From a simple Universal Data Model, Elsamadisi founded his own company to help startups use this approach so they could understand customer behaviour better. Source: George Frey/AFP
What were the practical learning elements in your course? Do you get to apply them in real life?
Definitely! I used the leadership theory in how I organise my company. I use the house of quality (a design tool of the management approach) to ensure our product delivers based on customer needs. I also use the kalman filter and particle filters in so much of my code. In terms of math, so much of what I do would fall under Bayesian mathematics (procedure with probabilities for statistical problems).
What skills or knowledge do you wish you had learned more during uni?
I would have loved to learn more on how to negotiate. I took some persuasion courses but it wasn’t enough. In sales, in meetings, and in raising money — there are a lot of negotiations that I wasn’t trained enough in.
I also wish we learned what the industry is recently using. Spending so much time learning engineering theories to practise them doesn’t mean there are things in the industry that are always done to get systems to work. Like 90% of all the physics equations I learnt, I later found out a simpler way for the approximation of reality.
Also data! We spent so much time in engineering but we do not learn how to store, manage or analyse data. Data analyses in school is so far from the truth that I would even consider it proper data analysis.
Do you have any advice for students planning to enrol in the same course as you?
Stop trying hard to get As! Aim for a B or B+ and spend the rest of your time in labs or with project teams. Focus more on applying what you have learned.
Also your professors are geniuses, so ask them questions that interest you instead of solutions to problems. I would ask my professors how a concept is used in a recent innovation and the learnings I got from these questions were priceless.
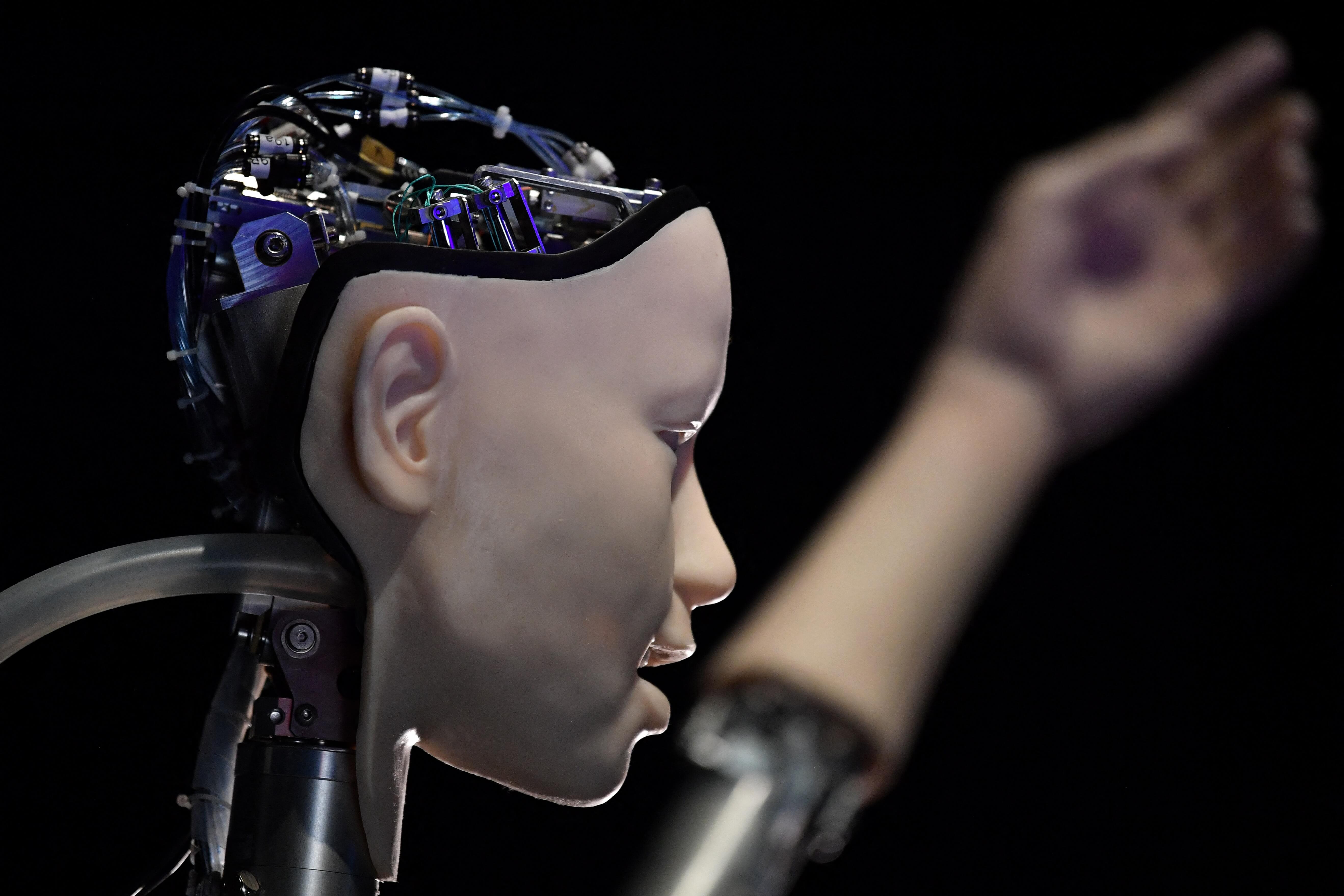
He once did a big project where he had robots play the game 20Q with many Cornell University students at the same time. Source: Ben Stansall/AFP
In 10 years, where would you like to be living and what would you like to be doing?
I will probably be location independent and spend a couple of months in each location so I can continue to move around the world. I am still working and will be making sure every person and company can benefit from the innovation of Narrator.
During your time researching robotics with enabling human-robot interactions, what were some of the things you discovered that stood out most?
There were two major learnings. One, you can make better decisions even using only data that is not accurate. For example, I did a big project where I had robots play the game 20Q with many Cornell University students at the same time. The twist was that people can lie or not know the answer. You would think that if you combine all this unreliable data would be worse off, but it turns out you can improve your ability to come to the right answer times five.